Best Terms Used to Describe Interprete Regression Result
R-sq adj Use adjusted R 2 when you want to compare models that have different numbers of predictors. Suppose the hypothesis needs to be tested for determining the impact of the availability of.
Dss Interpreting Regression Output
The example here is a linear regression model.
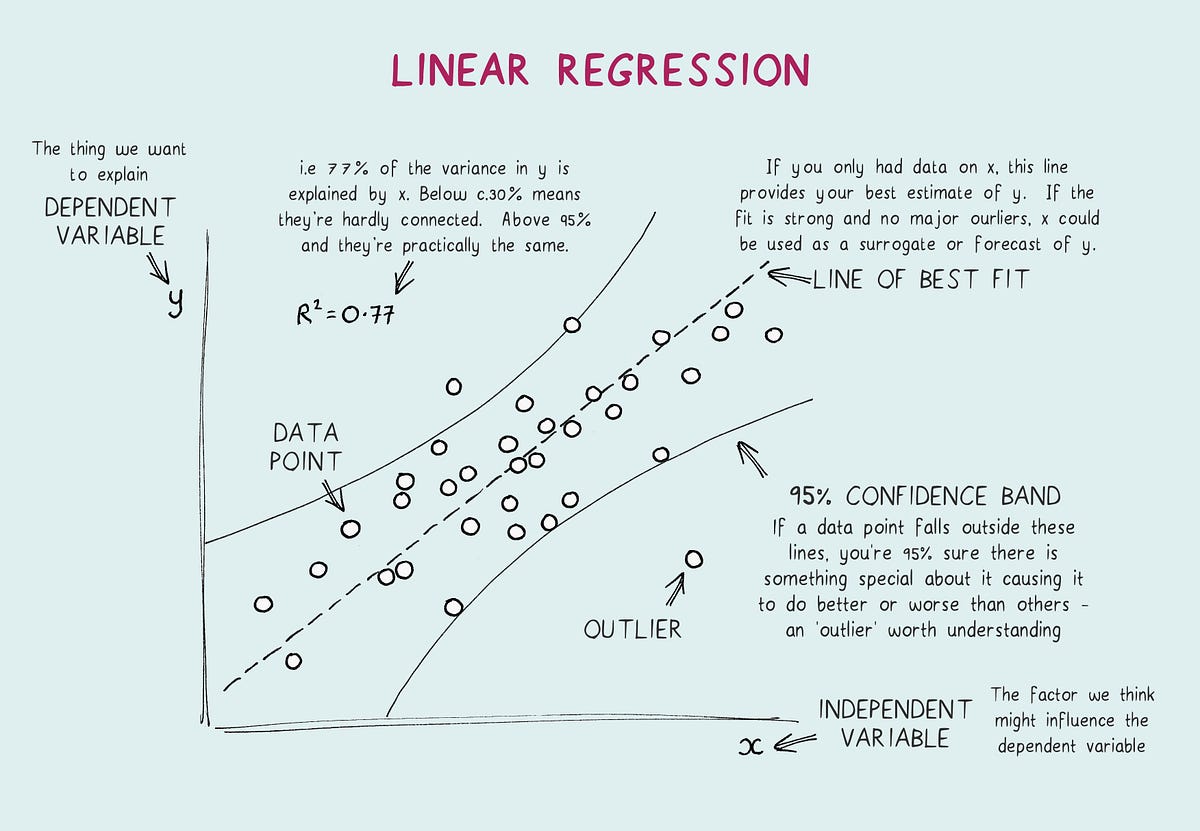
. Unlike a linear relationship the effect of the independent variable changes based on its value. This is the variation that is not explained by our regression model. The sign of the coefficient is positive which indicates that as density increases stiffness also increases.
Regression analysis that uses polynomials to model curvature can make interpreting the results trickier. The error sum of squares or SSE is a measure of the random error or the unexplained variation. We can use these coefficients to form the following estimated regression equation.
A P of 5 or less is the generally accepted point at which to reject the null hypothesis. The coefficients can be different from the coefficients you would get if you ran a univariate regression for each factor. The P value is the probability of seeing a result as extreme as the one you are getting a t value as large as yours in a collection of random data in which the variable had no effect.
Or you could consider log transformation 2 Significance of quadratic terms could signal that the relation is non-linear. This type of distribution forms in a line hence this is called linear regression. This is also referred to as sum of squared errors.
This tutorial explains how to interpret the intercept value in both simple linear regression and multiple linear regression models. Regression Equation Coefficient In these results the coefficient for the predictor Density is 35405. While interpreting the p-values in linear regression analysis in statistics the p-value of each term decides the coefficient which if zero becomes a null hypothesis.
In statistics regression analysis is a technique that can be used to analyze the relationship between predictor variables and a response variable. The independent variable s that will be used is are represented as X Regression is simply establishing a relationship between the independent variables and the dependent variable. Complete the following steps to interpret a regression analysis.
The overall regression was statistically significant R 2 73 F1 18 4799 p 000. A low p-value of less than 05 allows you to reject the null. It is one of the most common types of predictive analysis.
Instead graph the data to truly understand the relationship. First well take a quick look at the simple correlations. For each observation this is the difference between the response value and the predicted value.
Suppose a professor would like to use the. Regression analysis is one of multiple data analysis techniques used in business and social sciences. However you may not have studied these.
For each predictor variable were given the following values. 1 Adding quadratic terms allows for non-linearity in a linear model. The model for a multiple regression can be described by this equation.
Therefore R 2 is most useful when you compare models of the same size. Y i B 0 B 1 X 1i B 2 X 2i e i. Interpreting the Intercept in Simple Linear Regression.
This is done with the help of hypothesis testing. Key output includes the p. Linear regression is basically a statistical modeling technique which used to show the relationship between one dependent variable and one or more independent variable.
The regression analysis technique is built on many statistical concepts including sampling probability correlation distributions central limit theorem confidence intervals z-scores t-scores hypothesis testing and more. Example of Interpreting and Applying a Multiple Regression Model Well use the same data set as for the bivariate correlation example -- the criterion is 1st year graduate grade point average and the predictors are the program they are in and the three GRE scores. A linear regression model with two predictor variables results in the following equation.
Y the response variable. Learn some of the most common terms used in regression analysis. Linear regression is establishing a relationship between the features and dependent variable that can be best represented by a straight line.
The variables in the model are. If you think that the relation between your target variable and a feature is possibly non-linear you can add quadratic terms. Exam score 671617 52503hours studied.
Reporting Results of Multiple Linear Regression. Y β0 β1x1 β2x2 β3x3 ε Where y is the dependent variable x i is the independent variable and β i is the coefficient for the independent variable. This tells us the average increase in the response variable associated.
When trying to decipher the results of a regression analysis you must understand the lingo as well. The fitted regression model was. The best five-predictor model will always have an R 2 that is at least as high the best four-predictor model.
Ultimately it is up to you to decide whether the improved detail of the results presented in Figure 16 compared with those presented in Figure 15 is worth the. But this works the same way for interpreting coefficients from any regression model without interactions. It was found that hours studied significantly predicted exam score β 52503 p 000.
With a P value of 5 or 05 there is only a 5 chance that results you are seeing would have come up in a random. Looking at the coefficients wont make the picture any clearer. In this article we will take the examples of Linear Regression Analysis in Excel.
The average stiffness of the particle board increases by 35405 for every 1 unit increase in density. The intercept sometimes called the constant in a regression model represents the mean value of the response variable when all of the predictor variables in the model are equal to zero. Regression is a statistical technique to formulate the model and analyze the relationship between the dependent and independent variables.
This section displays the estimated coefficients of the regression model. For a linear regression analysis following are some of the ways in which inferences can be drawn based on the output of p-values and coefficients. When you use software like R Stata SPSS etc to perform a regression analysis you will receive a regression table as output that summarize the results of the regression.
Mpg 2939 03hp 162drat 323wt. It aims to check the degree of relationship between two or more variables. Regression lines based on weighted model 1 estimates are presented in Figure 15 whereas Figure 16 demonstrates the more nuanced story told by the estimates obtained by using model 2 which includes interaction terms.
Simple Linear Regression Examples Linear Regression Data Science Data Science Learning
Linear Regression Explained A High Level Overview Of Linear By Jason Wong Towards Data Science
No comments for "Best Terms Used to Describe Interprete Regression Result"
Post a Comment